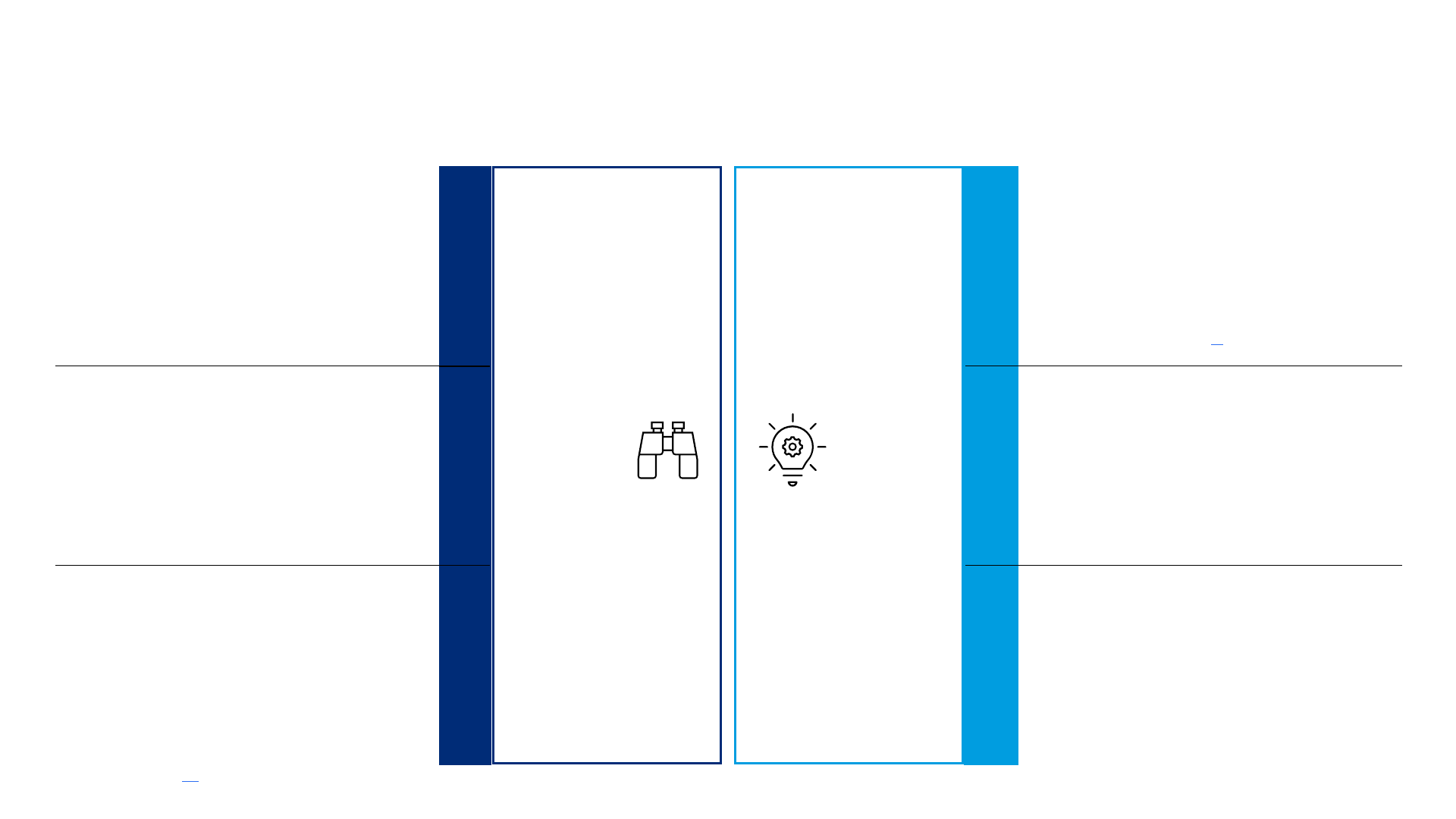
24
© Oliver Wyman
Descriptive
First round of analysis laid the
foundation of a structured
data collection framework.
It can now be updated to
enhance or refresh the
analysis data
Explanatory
Now that the current market
conditions are better
understood and the a priori
hypotheses have been tested,
additional research might be
of interest to understand the
underlying causes.
POSSIBLE ADDITIONAL AREAS OF RESEARCH
Findings stemming from this first analysis on the topic have sparked interest for additional analysis ideas on future iterations.
Timely Data Refresh
Insurance availability/affordability is a time-
sensitive topic that can take quick and significant
turns.
Depending on market signals, it might be beneficial
to have timely refreshes to assess the emerging
trends in 2023.
1
More Granular Exposure Data
Our first iteration was focused on creating a
streamlined process to obtaining structured and
accurate information from all carriers.
Now that this has been achieved, it might be
interesting to enhance the data collection to obtain
more targeted answers, for example by collecting
new business, renewal, and non-renewals data.
2
Inflation-adjusted metrics
The current high-inflationary environment certainly
has an impact on the premium movements
currently observed in the state.
It would be interesting to identify what share of
rate changes/premium increases is driven by
inflation vs other factors.
3
1
Exposure to Other Perils (e.g., Hail)
Our study has shown that the premiums and
exposure swings go beyond the regions at high risk
of wildfire, which highlights other types of
exposures may have played a role.
Hail risk might be an interesting area to examine,
given it has led to multi-billion dollars losses in the
state as recently as 2018.
[1]
2
Historical Profitability Within the State
The current study looked at historical profitability
at the state level in order to answer the most
pressing questions on the topic.
Given the results, in future iterations it might be
interesting to assess to which extent the
underwriting results vary across the geographic
regions of the state.
3
Exposures Growth and Housing Developments
To help understand the industrywide trends in
exposures by county and/or ZIP code, it would be
beneficial to obtain measurable data on the
number of new houses to insure in each region.
This would also allow to assess to which extent new
development are taking place in “high risk” areas.
Source: The Gazette [1]